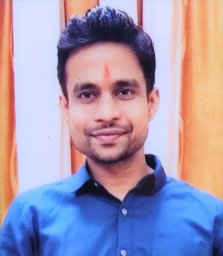
Ankit A. Bhurane
Department of Electronics and Communication, Visvesvaraya National Institute of Technology , India
Title: sleep disorders using convolutional recurrent neural network
Biography
Biography: Ankit A. Bhurane
Abstract
Healthy sleep is an essential criterion for a healthy life. However, people nowadays face many sleeping disorders, making their lives miserable. Hence, proper sleep monitoring is essential for detecting and diagnosing such sleep disorders. To address this issue, we can use the cyclic alternating patterns (CAP) to monitor the CAP phases, which tells us about the sleep quality and different disorders like insomnia, PLM, RBD, etc. This process of detecting CAP is time-consuming, hectic, and prone to errors. Therefore, there is a need to create an automatic detection of CAP phases for swift monitoring. A hierarchical approach to identify sleep disorders and classifying CAP sleep phases can be suitable in this regard. Single-channel EEG CAP sequence can be utilized to classify the data into healthy or unhealthy. Further, the sleep disorder of unhealthy sequence can be classified among rapid eye movement behavior disorder (RBD), narcolepsy (NARCO), periodic leg movement disorder (PLM), nocturnal frontal lobe epilepsy (NFLE), and insomnia (INS). Even further, the CAP phase of given sequence can be identified using our previous work. The best model for healthy, unhealthy, and disease classification can be obtained by long short-term memory (LSTM) and convolutional neural network (CNN). The same models, when evaluated using a dataset of only phase can give better accuracy for healthy-unhealthy classification and disease classification, respectively, indicating the significance of phase B for sleep disorder identification.